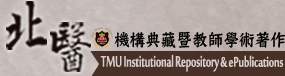 |
English
|
正體中文
|
简体中文
|
全文筆數/總筆數 : 45422/58598 (78%)
造訪人次 : 2541314
線上人數 : 186
|
|
|
資料載入中.....
|
請使用永久網址來引用或連結此文件:
http://libir.tmu.edu.tw/handle/987654321/65140
|
題名: | Clinical narrative-aware deep neural network for emergency department critical outcome prediction |
作者: | 張詠淳 Min-Chen Chen, Ting-Yun Huang, Tzu-Ying Chen, Panchanit Boonyarat, Yung-Chun Chang |
貢獻者: | 大數據科技及管理研究所 |
關鍵詞: | Emergency department;Prediction model;Clinical narrative;Critical care;Natural language processing;Deep learning |
日期: | 2023-02 |
上傳時間: | 2025-03-26 15:07:20 (UTC+8) |
摘要: | Since early identification of potential critical patients in the Emergency Department (ED) can lower mortality and morbidity, this study seeks to develop a machine learning model capable of predicting possible critical outcomes based on the history and vital signs routinely collected at triage. We compare emergency physicians and the predictive performance of the machine learning model. Predictors including patients’ chief complaints, present illness, past medical history, vital signs, and demographic data of adult patients (aged ? 18 years) visiting the ED at Shuang-Ho Hospital in New Taipei City, Taiwan, are extracted from the hospital’s electronic health records. Critical outcomes are defined as in-hospital cardiac arrest (IHCA) or intensive care unit (ICU) admission. A clinical narrative-aware deep neural network was developed to handle the text-intensive data and standardized numerical data, which is compared against other machine learning models. After this, emergency physicians were asked to predict possible clinical outcomes of thirty visits that were extracted randomly from our dataset, and their results were further compared to our machine learning model. A total of 4,308 (2.5 %) out of the 171,275 adult visits to the ED included in this study resulted in critical outcomes. The area under the receiver operating characteristic curve (AUROC) and the area under the precision-recall curve (AUPRC) of our proposed prediction model is 0.874 and 0.207, respectively, which not only outperforms the other machine learning models, but even has better sensitivity (0.95 vs 0.41) and accuracy (0.90 vs 0.67) as compared to the emergency physicians. This model is sensitive and accurate in predicting critical outcomes and highlights the potential to use predictive analytics to support post-triage decision-making. |
關聯: | Journal of Biomedical Informatics; 138; 104284 |
描述: | 【112-1 升等】臺北醫學大學教師升等專門著作 職別:專任 送審等級:教授 著作送審 |
資料類型: | article |
顯示於類別: | [教師升等送審著作] 112 [大數據科技及管理研究所] 期刊論文
|
文件中的檔案:
檔案 |
描述 |
大小 | 格式 | 瀏覽次數 |
index.html | | 0Kb | HTML | 1 | 檢視/開啟 |
|
在TMUIR中所有的資料項目都受到原著作權保護.
|