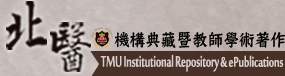 |
English
|
正體中文
|
简体中文
|
全文筆數/總筆數 : 45243/58419 (77%)
造訪人次 : 2486478
線上人數 : 222
|
|
|
資料載入中.....
|
請使用永久網址來引用或連結此文件:
http://libir.tmu.edu.tw/handle/987654321/64993
|
題名: | Advancing Genomic Analysis for Antimicrobial Resistance Prediction: Pan-Genome Insights and Robust Machine Learning Approaches |
作者: | DUYEN, DO THI |
貢獻者: | 醫學資訊研究所博士班 吳育瑋 |
關鍵詞: | 細菌抗藥性;Unitig;de Bruijn圖;單位點變異;基因叢集;泛基因體;基因演算法;特徵選取;綠膿桿菌 Antimicrobial resistance;Unitig;de Bruijn graph;SNP;Gene cluster;Pan-genome;Genetic Algorithm;Feature selection;Pseudomonas aeruginosa |
日期: | 2024-06-19 |
上傳時間: | 2025-01-06 09:13:32 (UTC+8) |
摘要: | 最近這幾十年來,全球的細菌抗生素抗藥性都在增加中,而施用抗生素的失效率也越來越高。我們因此急切需要準確且快速的細菌抗藥性防治方案。雖然使用機器學習演算法透過基因進行抗藥性預測的作法相當普遍,但大部分既有的方法都是透過已知的抗藥性基因進行預測。然而細菌抗藥性的機制仍在持續發現中,而這種透過已知基因進行預測的作法無法讓我們找到新的抗藥性基因,或是將新的抗藥性基因加入預測模型中提高預測準確率。在本學位論文中,我提出了透過細菌泛基因體進行機器學習模型架構的做法。我還將探索各種不同的泛基因體建構方法(包括Unitig以及不同的基因表示方法)是否會影響到抗藥性預測的準確率。 在論文的第一部分,我建構了以Unitig為主體的泛基因體。Unitig是透過Compact de Bruijn graph(簡寫為cDBG)建構而成的主要單位,而泛基因體即是將cDBG方法套用在上千株綠膿桿菌(Pseudomonas aeruginosa)後得出的Unitig出現或未出現(Presence/Absence)在這些綠膿桿菌菌株上的分布。我發現將機器學習演算法套用在這個Unitig泛基因體上可以得到相當好的預測準確率。不只如此,我還將特徵選取演算法套用在泛基因體上以達到更好的預測準確率,而演算法選出的特徵集還可以讓我進一步分析選出的Unitig上的抗藥性基因分布。 而在論文的第二部分,我試著透過不同的方法建構以基因為主體的泛基因體。與前述以Unitig為主體的泛基因體最大的不同點,在於以基因為主體的泛基因體探究的是基因在不同菌株中的分布。不只如此,我還試著從基因中萃取出單位點變異(Single Nucleotide Polymorphism;SNP)資訊,並建構出另一個泛基因體。我還將基因分布與單位點變異分布這兩個資訊合併起來,形成第三個泛基因體。我比較了這三種不同的泛基因體對抗藥性預測的效能,結果顯示將兩種不同的資訊合併起來的泛基因體有著最好的預測功效。我還開發出了以基因演算法(Genetic Algorithm)為主體的特徵選取演算法,並透過它選出最能夠用來預測抗藥性的基因,以提高抗藥性預測的準確率。 總的來說,在這篇論文中我探索了不同的泛基因體細菌抗藥性預測模型,並透過特徵選取演算法同時達到提高預測準確效能以及模型解釋與分析這兩個目的。我期望我提出的機器學習特徵選取演算法能夠在未來更進一步地用在降低模型複雜度,並更完善地結合資料與預測目標;而我的抗藥性預測模型則能夠用來更完整地分析細菌的抗生素抗藥性機制。 Antimicrobial resistance (AMR) poses a critical global health challenge and needs swift and accurate diagnostic solutions. Despite the popularity of machine learning methods in AMR detection for their adeptness with complex datasets, existing approaches often focus on well-documented resistant genes or databases, limiting their ability to identify novel AMR elements. To overcome these limitations, this dissertation proposes pan-genome-based machine learning approaches to enhance our understanding of AMR gene repertoires and uncover potential feature sets for precise AMR classification. Using whole genome sequencing data of Pseudomonas aeruginosa strains, various types of pan-genomes were constructed, including unitig-centered and gene-based pan-genomes. The gene-based pan-genomes were further divided into gene cluster-based and SNP-based pan-genomes. These pan-genomes were investigated to explore their capabilities predicting AMR and extracting potential resistance genes. In the first part of the thesis, I constructed the unitig-centered pan-genome using compact de Brujin graph (cDBGs) from thousands of genomes and collected presence/absence patterns of unique sequences (unitigs) for Pseudomonas aeruginosa. By applying machine learning models on the unitig-centered pan-genome, I found that the AMR phenotypes can be predicted accurately, indicating the usefulness of the unitig-centered pan-genome. The application of feature selection model on the pan-genome not only boosts the prediction accuracy but also allows the investigation of potential AMR genes on the selected unitigs. In the second part of the thesis, I investigated the gene-based pan-genome from two different aspects, namely gene cluster-based, SNP-based, and a combined approach incorporating both gene presence/absence patterns and SNP information. A two-step feature selection-based genetic algorithm (GA) further was developed to identify significant features for AMR prediction across these pan-genomes. Systematic comparison revealed that the combined pan-genome approach outperformed the individual methods, highlighting its superiority as an AMR predictor. Moreover, the proposed GA feature selection method effectively identified highly relevant features for AMR prediction, resulting in a significant improvement in the F1-score and a substantial reduction in the number of features. Through the exploration of pan-genome applications in predicting AMR, I successfully develop not only accurate but also explainable machine learning predictors, which could help uncover the underlying mechanisms of AMR. I hope my research could help advance genome representation techniques in reducing data complexity and enabling models to more accurately capture the relationship between the data and AMR phenotypes. |
描述: | 博士 指導教授:吳育瑋 口試委員:黎阮國慶 口試委員:蘇家玉 口試委員:張家銘 口試委員:郭朝揚 口試委員:吳育瑋 |
附註: | 論文公開日期:2024-07-02 |
資料類型: | thesis |
顯示於類別: | [醫學資訊研究所] 博碩士論文
|
文件中的檔案:
檔案 |
描述 |
大小 | 格式 | 瀏覽次數 |
index.html | | 0Kb | HTML | 132 | 檢視/開啟 |
|
在TMUIR中所有的資料項目都受到原著作權保護.
|