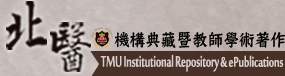 |
English
|
正體中文
|
简体中文
|
全文筆數/總筆數 : 45422/58598 (78%)
造訪人次 : 2511954
線上人數 : 273
|
|
|
資料載入中.....
|
請使用永久網址來引用或連結此文件:
http://libir.tmu.edu.tw/handle/987654321/63345
|
題名: | 使用深度學習於 Hep-2 Cell 影像分型輔助自體免疫疾病診斷 Automated Hep-2 Cell Image Classification to Support Autoimmune Disease Diagnosis by Deep Learning Approach |
作者: | 劉于嘉 LIU, YU-JIA |
貢獻者: | 醫學院人工智慧醫療碩士在職專班 邱泓文 |
關鍵詞: | 深度學習;影像辨識模型 deep learning;image classification model |
日期: | 2023-07-14 |
上傳時間: | 2023-12-15 14:37:19 (UTC+8) |
摘要: | 抗核抗體檢驗(Anti-nuclear autoantibodies test, ANA test)是自體免疫疾病作為重要的篩檢工具,其中辨識抗核抗體型態較耗費人力,且容易有主觀判讀造成報告不一致的問題。本研究主要目標為以公開的pre-trained CNN model建構出能辨識混合抗核抗體型態的高準確度模型,其表現能與臨床上資深醫檢師的辨識結果有高度一致性,本研究次要目標為探討直接以常見的混合型態和單一型態作為模型分辨的種類,和過去研究當中使用多標籤分類法的模型設計方式相較,是否模型的影像辨識表現可以提升。 其檢驗原理為間接免疫螢光法(Indirect immunoFluoresence Assay; IFA),在螢光顯微鏡下觀察加入病人血清並且染色後的Hep-2 cell的螢光訊號強度與分布,判讀病人血清當中是否有自體免疫抗體,並依據Hep-2 cell螢光在細胞分布狀況,判斷抗核抗體型態(Antinuclear Antibody (ANA) Patterns, 以下簡稱為ANA型態)。臨床上ANA型態經常為混合型態,所以現實上經常是影像判讀系統提供的答案,醫檢師仍需逐張檢視確認,將系統遺漏判讀的型態補上報告。 為更符合臨床上的應用,本研究共蒐集2020年1月至2023年5月以來雙和醫院醫學檢驗科ANA test留存共1894張圖檔作為模型的資料集,將圖檔整理出包含單一型態與混合型態共11類,利用深度學習以pre-trained CNN model, InceptionResNetV2建構能辨識ANA混合型態之模型,並且在訓練過程中以交叉驗證 K-Fold Cross Validation(k=5)作為驗證訓練表現。在測試集分為兩個部分,分別用於評估模型表現,以及所提出之模型與一位有資深經驗的醫檢師和兩位初學者進行ANA型態之辨識,結果將與所提出之模型作一致性評估。 本研究結果建置之模型效能表現mean class accuracy(MCA)最高達成87.8%、F1 score可達87.8%。評估個別種類分類效能的表現分析,其中混合型態之類別Accuracy的表現較差,分析可能有兩個原因:混合型態的資料量相對其他類別較少,可能造成模型學習成效不佳。第二原因:為混合型態包含兩項特徵,其特徵表現比重較偏頗於其中一邊時可能就會造成誤判。關於AI模型與醫檢師的一致性測驗,AI模型與資深醫檢師Kappa係數達85.5%,說明AI模型同資深醫檢師在ANA影像辨識有不錯的一致性,且AI模型的表現優於初學者(83.6% vs 65.5%),說明對於初學者來說,在臨床學習過程中,AI模型也許能達到輔助的效果。 Anti-nuclear autoantibodies test (ANA test) is an important screening tool for autoimmune diseases. Identifying the type of anti-nuclear antibodies is labor-intensive and poor agreement in reports due to subjective interpretation. The main goal of this study is to construct a high-accuracy model that can identify the types of mixed antinuclear antibody patterns based on the pre-trained CNN model, and also achieved an excellent agreement with senior medical technologists. The second goal is to investigate whether the performance of this model was more excellent than those models using multi-label classification method in previous research. The principle of ANA test is based on indirect immunofluoresence Assay (IFA). The intensity and distribution of the fluorescent signal of human epithelial cells (HEp-2) cell added to the patient serum and stained under a fluorescent microscope are observed to determine the antinuclear antibody pattern (ANA pattern) according to the distribution of fluorescence in the Hep-2 cell. In clinical practice, ANA patterns are often mixed. While image interpretation systems provide answers, medical technologists still need to review and confirm each image, adding any missed patterns to the report. In order to be more in line with clinical applications, 1894 HEp-2 cell images with patterns assigned by experienced medical technologists collected in the Shuang Ho Hospital from January 2020 to May 2023 as the dataset. A dataset containing 11 classes of single and mixed ANA patterns was used to train a deep learning model using InceptionResNetV2. K-Fold Cross Validation (k=5) was used during training for performance validation. The testing set was divided into two parts: one for evaluating the model's performance and the other for assessing its consistency with a senior medical technologist and two beginners in identifying ANA patterns. The performance of the proposed model in this study achieved a mean class accuracy (MCA) of 87.8% and an F1 score of up to 87.8%. In the analysis of individual class classification performance, the accuracy in mixed patterns showed poorer performance. This can be attributed to two possible reasons. First, the data of mixed patterns is relatively smaller compared to other classes, which may have resulted in less effective learning by the model. The second reason is that mixed patterns contain two distinct features, and if the model's performance is biased towards one feature, it can lead to misclassifications. Regarding the consistency test between the AI model and experienced medical technologist, the Kappa coefficient reached 85.5%, indicating a good agreement in ANA image recognition between the AI model and the experienced medical technologist. Furthermore, the AI model outperformed beginners, achieving 83.6% accuracy compared to 65.5%. This suggests that the AI model could potentially serve as a helpful tool in assisting beginners during their clinical learning process. |
描述: | 碩士 指導教授:邱泓文 委員:彭徐鈞 委員:劉文德 委員:邱泓文 |
資料類型: | thesis |
顯示於類別: | [人工智慧醫療碩士在職專班] 博碩士論文
|
文件中的檔案:
檔案 |
描述 |
大小 | 格式 | 瀏覽次數 |
index.html | | 0Kb | HTML | 77 | 檢視/開啟 |
|
在TMUIR中所有的資料項目都受到原著作權保護.
|