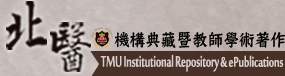 |
English
|
正體中文
|
简体中文
|
全文筆數/總筆數 : 45422/58598 (78%)
造訪人次 : 2514293
線上人數 : 197
|
|
|
資料載入中.....
|
請使用永久網址來引用或連結此文件:
http://libir.tmu.edu.tw/handle/987654321/62459
|
題名: | 基於自動化腦電波及心率變異訊號之慢波睡眠預測模型 Prediction Model of Slow Wave Sleep Based on Automatic EEG and HRV Classification |
作者: | 黃世友 HUANG, SHIH-YU |
貢獻者: | 醫學院人工智慧醫療碩士在職專班 劉文德 |
關鍵詞: | 慢波睡眠;心率變異性;機器學習 Slow wave sleep;Heart rate variability;Machine learning |
日期: | 2022-07-11 |
上傳時間: | 2023-01-17 14:52:49 (UTC+8) |
摘要: | 一、研究之背景與目的 慢波睡眠(slow wave sleep)在睡眠週期中屬深層睡眠的階段,這個階段的腦電波相較於其他睡眠階段會出現較高比例的delta waves (亦稱為slow waves)。此睡眠階段與身體能量的恢復、記憶鞏固、神經代謝產物的清除等過程有重大的關聯,為睡眠中重要的階段。然而,評估睡眠中的慢波睡眠的情形,需接受睡眠多項生理檢查(Polysomnography, PSG),過程需花上一定的時間、人力物力和繁複的分析判讀過程,執行上較為困難,也因此減少睡眠障礙者檢測的意願。再者,受限於睡眠期別的定義,在傳統睡眠評估報告中慢波睡眠腦電波的主角slow wave (亦即delta wave) 實際所佔的比例與時間長度是浮動而不確定的,故對於準確反映睡眠腦電波中slow wave的分布情形會有所限制。在本研究中,我們試圖另闢蹊徑,發展獲取及預測睡眠週期中真實慢波睡眠分布情形較簡便且可靠的方式,建立使用心率變異性參數等資訊為特徵,以預測慢波睡眠組成狀況之機器學習及深度學習模型,預測於5分鐘睡眠區段中慢波睡眠時間佔比之分類。 二、研究方法 以回溯性的方式收集使用55位雙和醫院接受睡眠多項生理檢查的記錄,將資料進行每5分鐘為一段之分割後,提取共3769筆資料之時間軸、腦電圖及心電圖之訊號進行分析。先將腦電圖之訊號,使用自動化腦電波分析之Python工具箱,定義計算出慢波(slow wave)之區段及總時長,經轉換為慢波時間佔比(slow-wave ratio)後,以慢波時間佔比5%或10%為標準,將慢波時長佔比多寡做二元分類及三元分類,共四種分類方式。接著,再以心率變異性之參數搭配人口統計變項作為特徵,經由機器學習及深度學習的方式,建立及訓練針對睡眠週期中慢波睡眠佔比類別之預測模型,並評估成效。 三、結果 使用心率變異性參數及受測者之年齡、性別、BMI作為特徵,透過機器學習及深度學習訓練出的預測模型,具備分類慢波時長佔比類別的能力。在區分慢波時長佔比最高與最低兩類之二元分類模型有最好的分類效果Accuracy:90%,ROC AUC:0.903,Cohen’s kappa:0.496。另外在其他二元分類及三元分類之模型也都具備分類慢波時長佔比類別的能力。 四、結論 本研究所訓練之模型在慢波時長佔比做二元分類時,皆能有效地以心率變異性之參數為主要特徵,預測5分鐘睡眠區段中慢波睡眠總時長佔據比例的類別,尤其在區分慢波時長佔比小於5%以及大於等於10%兩類時有最佳之分類效果,此研究結果有利於簡化評估慢波睡眠的流程及推進後續心率變異分析及慢波睡眠之相關研究。 Background and Objective: Slow wave sleep is an important stage of deep sleep in the sleep cycle, and electroencephalographic (EEG) in this stage consists of a higher proportion of delta waves than other sleep stages. Condition of slow wave sleep is typically evaluated through polysomnography (PSG). However, PSG requires a certain amount of time, manpower and material resources, as well as a complex analysis and interpretation process, which is difficult to implement, and therefore it reduces the willingness of people with sleep disorders to detect. Based on the evidence that Heart rate variability (HRV) would change through the sleep cycle, this study developed new methods for assessing the composition of slow wave sleep in the sleep cycle. We trained and created a predicting model of slow wave sleep based on the automated EEG and HRV classification. Methods: Data were retrospectively collected from 55 participants who underwent polysomnography (PSG) in Shuang-Ho Hospital, Taiwan. The time axis, EEG signals and electrocardiogram signals (ECG) data were extracted from PSG records for analysis. After dividing the PSG records into 5-min epochs, we used automatic EEG analysis python toolbox to locate the segments of slow waves in every epoch. Later, the total duration and percentage of slow waves were computed for every epoch as the target of further prediction. Using the parameters of HRV and demographic variables as features, prediction models were trained by machine learning and deep learning to predicting the proportion classification of slow-waves ratio in every 5-min epoch during sleep. The performance of prediction models was evaluated. Results: In our study, the trained prediction models could successfully predict the class of slow-wave ratio (percentage) based on HRV parameters and demographic variables. The best performance could achieve an accuracy of 90 %, a Cohen’s Kappa of 0.496 with an AUROC of 0.903 in binary classification models. The prediction model for 3-class classification of slow-wave ratio also showed ability of classification with an AUROC of 0.713. Conclusion: Based on the performance of the prediction models of slow-wave ratio trained and tested in this study, the composition of slow wave in a 5-min epoch of sleep cycle can be effectively predicted by using HRV parameters and demographic variables as features, especially in binary classification models. The overall results are beneficial to simplifying the process of evaluation slow wave sleep and promoting the subsequent research on HRV and slow wave sleep. |
描述: | 碩士 指導教授:劉文德 委員:劉文德 委員:彭徐鈞 委員:何淑娟 |
資料類型: | thesis |
顯示於類別: | [人工智慧醫療碩士在職專班] 博碩士論文
|
文件中的檔案:
檔案 |
描述 |
大小 | 格式 | 瀏覽次數 |
index.html | | 0Kb | HTML | 155 | 檢視/開啟 |
|
在TMUIR中所有的資料項目都受到原著作權保護.
|