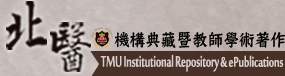 |
English
|
正體中文
|
简体中文
|
全文筆數/總筆數 : 45422/58598 (78%)
造訪人次 : 2514048
線上人數 : 146
|
|
|
資料載入中.....
|
請使用永久網址來引用或連結此文件:
http://libir.tmu.edu.tw/handle/987654321/61494
|
題名: | 運用深度學習技術分析時序性電子醫療紀錄以預測乾癬性關節炎風險 Predicting the Risk of Psoriatic Arthritis with Chronological Electronic Medical Records: A Deep Learning Approach |
作者: | 李宗儒 LEE, TSUNG-JU (LEON) |
貢獻者: | 醫學資訊研究所碩士班 李友專 |
關鍵詞: | 乾癬;乾癬性關節炎;時序表現型圖;機器學習;深度學習;卷積神經網絡;風險預測模型 Psoriasis;Psoriatic Arthritis;Temporal Phenomic Map;Machine Learning;Deep Learning;Convolutional Neural Network;Prediction Model |
日期: | 2021-07-25 |
上傳時間: | 2022-03-25 16:46:59 (UTC+8) |
摘要: | 研究背景: 乾癬是全身性的慢性發炎免疫疾病,常合併其他共病,像是代謝症候群與心血管疾病等,最常見的是乾癬性關節炎,約佔乾癬患者中的三成,其中15.5%未被正確診斷。若是能早期診斷乾癬性關節炎或預測高風險族群並積極介入治療將能減緩後續不可逆轉的關節變形與預防病患未來活動功能的喪失。目前臨床上尚未有良好的風險預測工具能協助醫師及早發現易罹患乾癬性關節炎的高風險族群。
研究目的: 利用全國性的電子醫療紀錄與機器學習演算法建立乾癬性關節炎的風險預測模型。
研究方法: 數據資料來源是臺灣全民健康保險研究資料庫隨機抽取的200萬患者(1999年1月1日至2013年12月31日),其中1,443位為乾癬性關節炎患者(ICD9 696.0)。本研究使用2.5年的門診與住院醫療資訊,建立包含診斷與藥物記錄的時序表現型圖。運用深度學習方法中的卷積神經網絡建立乾癬性關節炎風險預測模型,用以預測未來半年的罹病風險。模型建立後使用觀察遮蔽分析方法進行模型可解釋性研究,探討對於模型預測能力影響較大的臨床特徵以理解模型如何做出預測和決策。
研究結果: 本研究建立二個風險預測模型,第一個模型以1,443位乾癬性關節炎病患和5,772位非乾癬與非乾癬性關節炎病患隨機配對訓練模型與驗證表現。第二個模型以443位發病於乾癬之後的乾癬性關節炎病患與1,772位無合併乾癬性關節炎的乾癬病患隨機配對。第一個模型經過測試資料驗證,預測半年後乾癬性關節炎風險的準確度達到0.88、靈敏度0.77,特異性為0.92,ROC曲線下面積(AUROC)平均為0.80。第二個模型達到準確度0.64、靈敏度0.80,特異性為0.60,ROC曲線下面積(AUROC)平均為0.70。觀察遮蔽分析方法顯示自體免疫疾病、糖尿病、高血壓等已知乾癬相關共病與乾癬治療藥物會影響模型的預測能力。然而,與乾癬病理機轉不同的疾病,例如異位性皮膚炎,對於模型預測也有所貢獻。
研究結論: 本研究以卷積神經網路根據時序性電子醫療紀錄建立的風險預測模型可以預測半年後潛在的乾癬性關節炎病患,尤其是已經罹患乾癬的患者。協助醫療人員和醫療政策制定者針對乾癬性關節炎的高風險病患提供及早的檢查與治療,以避免未來更嚴重與不可逆的關節傷害產生。 Background: Psoriasis (PsO) is a chronic, systemic, immune-mediated disease with multiorgan involvement. Psoriatic arthritis (PsA) is an inflammatory arthritis that is present in 6%–42% of patients with PsO. Approximately 15% of patients with PsO have undiagnosed PsA. Predicting patients with a risk of PsA is crucial for providing them early examination and treatment that can prevent irreversible disease progression and function loss.
Objectives: Develop and validate a prediction model for PsA in patients with or without PsO based on chronological large-scale and multidimensional electronic medical record data by using a machine learning algorithm.
Materials and Methods: Data were obtained from Taiwan’s National Health Insurance Research Database (January 1, 1999 to December 31, 2013). A total of 1,443 patients with PsA (International Classification of Diseases, Ninth Revision, Clinical Modification code 696.0) were included in the analysis. A convolutional neural network was used to develop two risk prediction models by using 2.5 years of clinical diagnostic information and medical records (inpatient/outpatient) to predict the risk of PsA for a given patient within the next 6 months.
Results: The 6-month PsA risk prediction model, including a total of 1,443 patients with PsA and 5,772 without PsO or PsA, using sequential diagnostic information and drug prescription information as a temporal phenomic map could attain an accuracy of 0.88 and AUROC of 0.80. Another model included a total of 443 PsA patients with earlier diagnosis of PsO and 1,772 PsO patients without PsA, achieving an accuracy of 0.64 and an AUROC of 0.70. Negative predictive values of both models could achieve 0.93.
Conclusions: The findings of this study suggest that a risk prediction model can predict patients with a high risk of PsA. This model may help health care professionals and policy makers to prioritize treatment to target high-risk populations. |
描述: | 碩士
指導教授:李友專
委員:許明暉
委員:林明錦 |
資料類型: | thesis |
顯示於類別: | [醫學資訊研究所] 博碩士論文
|
文件中的檔案:
檔案 |
描述 |
大小 | 格式 | 瀏覽次數 |
index.html | | 0Kb | HTML | 1053 | 檢視/開啟 |
|
在TMUIR中所有的資料項目都受到原著作權保護.
|